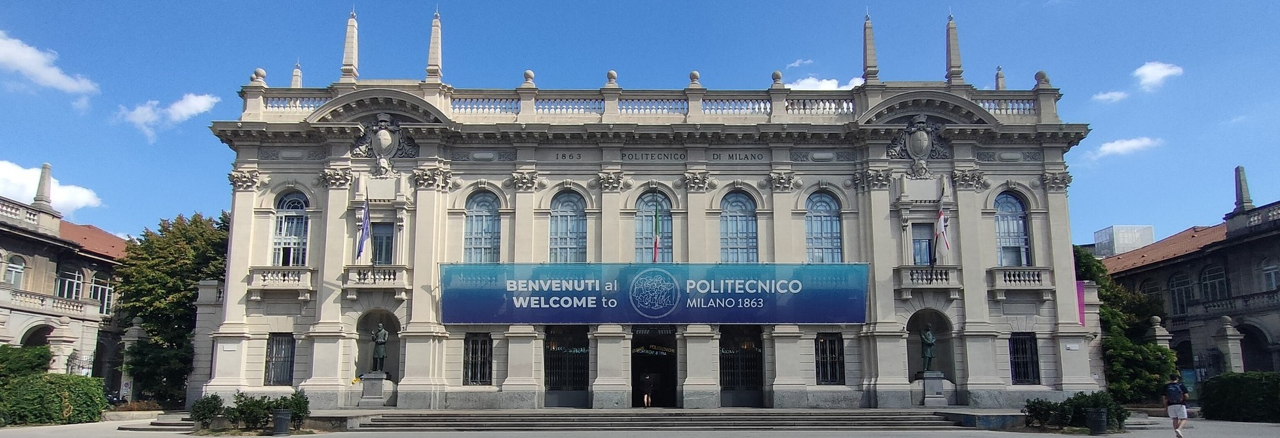
Our Research Areas
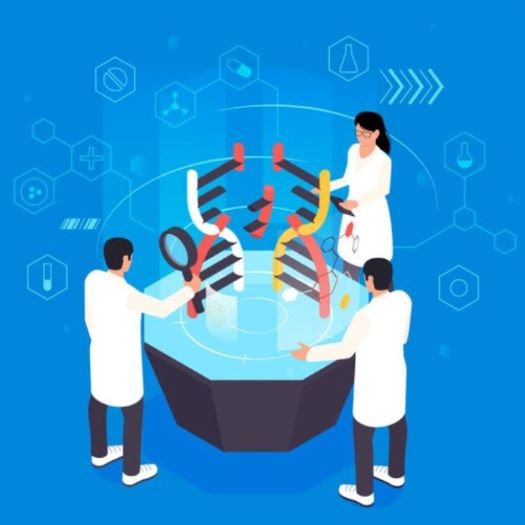
Artificial Intelligence in Health Care
Develop advanced analytical approaches and tools for improving diagnostics, treatment planning, and precision medicine.
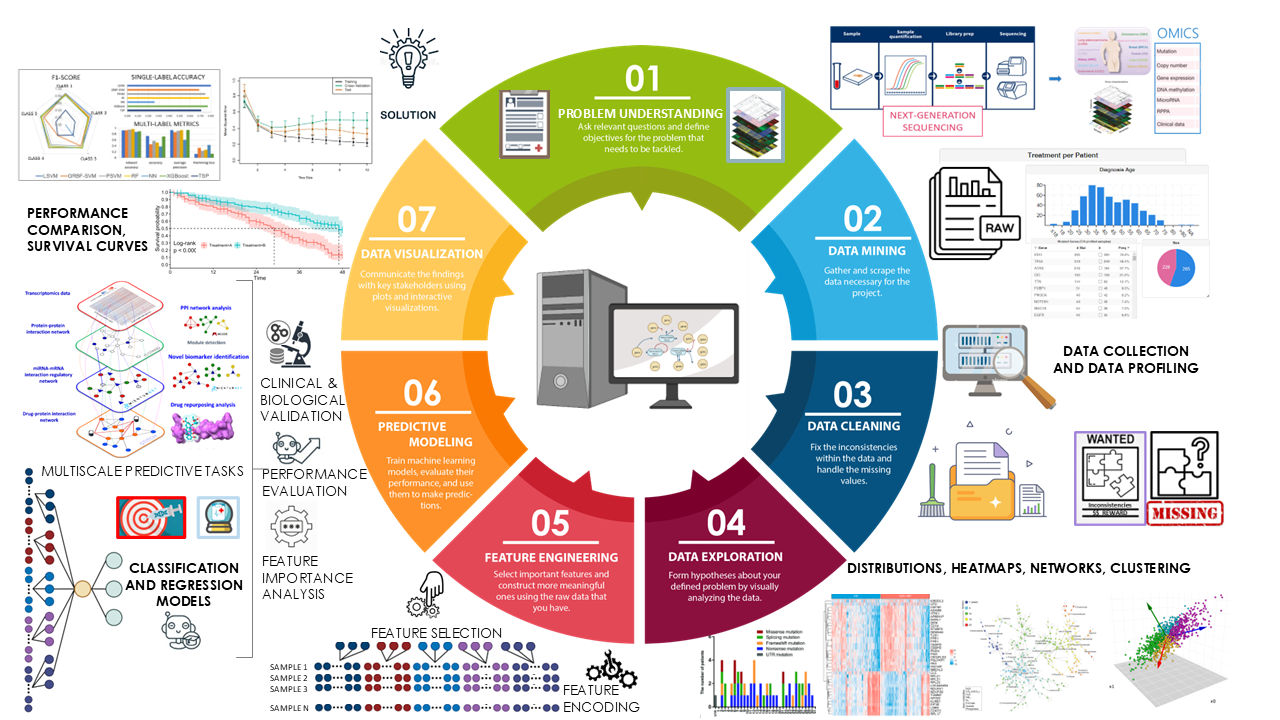
Omics Data Science
Leverage and design computational solutions to investigate large-scale omics data, and uncover insights to advance understanding of biological phenomena and diseases.

Computational Methods for Bioinformatics
Develop computational algorithms and tools to analyze heterogeneous data and interpret biological phenomena in bioinformatics.
Within these research areas, we integrate bioinformatics knowledge, mathematical and statistical data modelling, computer science principles, data analytics and machine learning approaches.
Explore our GitHub repository:
Projects and Funded Activities
Co-Funded by: Foundation IRCCS Neurology Institute “Carlo Besta” & National Recovery and Resilience Plan (PNRR)
A precision medicine approach based on machine learning and multiple molecular biology, biophysics, and clinical features will be developed to stratify ALS/FTD patients. The main algorithms for the analysis of high-throughput molecular and clinical data will be used to integrate and evaluate all available information to computationally answer complex research and clinical questions.
Collaborators: MIND lab, Neurological Institute ‘Carlo Besta’
Funded by: National Recovery and Resilience Plan (PNRR) Innovation Ecosystem
The biodiversity of aquatic ecosystems is a rapidly evolving field of research, and metabarcoding techniques are becoming increasingly important for studying the composition of biological communities within these ecosystems. However, the analysis of environmental data requires advanced computational approaches to manage the complexity and volume of the collected data. This project analyzes two environments, one fluvial and one marine, using metabarcoding techniques and developing automated protocols for data analysis, including machine learning approaches.
Collaborators: BMR Genomics Srl
Co-Funded by: Foundation IRCCS Neurology Institute “Carlo Besta” & National Recovery and Resilience Plan (PNRR)
Chronic pain is a complex disease characterized by the interaction between multiple gene variants, rare mutations, environmental factors, lifestyles, comorbidities, viral infections, etc. We leverage modern data science approaches and machine learning analysis to capture the heterogeneity of the disease and unravel its heritability. State-of-the-art machine learning models are used and analyzed with eXplainable AI strategies to evaluate the importance of genetic variants in the stratification of chronic pain patients, as well as to associate genetic and deep phenotypic and clinical traits, possibly leading to new relevant knowledge.
Collaborators: MIND lab, Neurological Institute ‘Carlo Besta’
Funded by: Research Project of National Relevant Interest (PRIN) – Italian Ministry of Education, Universities and Research
Computational analysis of RNA-seq data of colorectal cancer patient-derived and cell line-derived mouse xenografts, focused on ligand/receptor pairs and the “secretome”, to generate an interaction map depicting consistency, heterogeneity and significance of candidate paracrine interactions in colorectal cancer.
Collaborators: Oncogenomics lab, Candiolo Cancer Institute
Funded by: Research Project of National Relevant Interest (PRIN) – Italian Ministry of Education, Universities and Research
SENSIBLE will create an integrated framework for genomic surveillance of viral pathogens, utilizing data-driven and knowledge-based analyses. By leveraging knowledge from COVID-19, it seeks to enhance understanding of viral pathogens and aid healthcare decision-making.
https://sensible-prin.github.io/
Collaborators: Università degli Studi di Milano
Co-Funded by: Pharmacological Research Institute “Mario Negri” IRCCS & National Recovery and Resilience Plan (PNRR)
Computational genomics methods for the repurposing of valproic acid and simvastatin in the treatment of pancreatic carcinoma. The research aim to study the synergistic antitumor interaction between valproic acid and simvastatin and its potentiation of gemcitabine/nab-paclitaxel doublet chemotherapy in patients with metastatic pancreatic adenocarcinoma. We analyze transcriptomic tumor data under different combinatorial treatments to find differentially expressed genes and pathways that might underline the mechanism of action of this synergy.
Collaborators: Pharmacogenomics Unit, Pharmacological Research Institute “Mario Negri”
Funded by: Telethon Foundation ETS
Developing computational methods for the characterization of cell-free epigenome in human diseases and gene therapy treated patients, and for clonal tracking in patients under gene therapy and stem cell transplantation. Particularly, new bioinformatics methods will be developed to fully characterize vector integrations and deconvolute cell-free DNA composition, both of which are crucial for assessing the safety and efficacy of gene therapy treatments. Furthermore, leveraging genomic somatic mutations in vivo, which have been used to characterize cells, tumour progression and aging, we will develop a novel method to quantify the proliferation stress in transplanted stem cells based on tracking somatic mutations over time in gene therapy patients.
Collaborators: Telethon Institute for Gene Therapy – San Raffaele Hospital
Funded by: National Recovery and Resilience Plan (PNRR) Innovation Ecosystem
Development of an innovative digital platform for fast and secure storage and exchange of big data in life sciences, translate basic research into applications aiming to improve nutritional, healing and environmental conditions of citizens, organize data collection and processing with Artificial Intelligence techniques. Particular focus on the development of computational approaches to enhance the biological interpretability of classification and feature selection algorithms and to improve patients’ stratification in supervised and unsupervised scenarios, with the aim of unlocking the secrets hidden in genes for better-personalized healthcare and treatment decisions towards improved precise medicine.
Collaborators: University of Milan-Bicocca, School of Medicine and Surgery
Co-Funded by: Italian Ministry of Health – Alliance Against Cancer (ACC) IRCCS Oncological Network
Development and application of analytical approaches of computational onco-genomics, through machine learning and deep learning techniques, within the research project: “Feasibility study for the joint genomic diagnosis of genetic risk and sensitivity to new drugs in breast, ovary and colon cancer” (GerSom) funded by the Italian Ministry of Health.
Collaborators: Oncogenomics lab, Candiolo Cancer Institute
Funded by: Pharmacological Research Institute “Mario Negri” IRCCS / IRCCS Humanitas Research Hospital
Developing computational and bioinformatics methods for the characterization of cancer genomic and transcriptomic profiles obtained through Next-Generation Sequencing (NGS) technology, to advance cancer research as well as patient care and outcomes. Focusing on Ovarian Cancer, specifically on high-grade serous ovarian tumours, we will apply the developed methods to find potential biomarkers and improve early diagnosis and treatment response prediction.
Collaborators: Pharmacological Research Institute “Mario Negri” and Humanitas Research Hospital
Funded by: European Institute of Technology (EIT)
Development of a computational system for the collection and bioinformatics analysis of viral sequences and their related information, available in multiple heterogeneous data sources.
Funded by: Piemonte Foundation for Oncology IRCCS
Development of analysis methods for genomic gene expression data through machine learning, within the research project: “STRA-RNA” funded by the Italian Ministry of Health – Alliance Against Cancer (ACC) IRCCS Oncological Network
Collaborators: Oncogenomics lab, Candiolo Cancer Institute
Funded by: European Research Council (ERC)
Development of an open source system, available for the biological and clinical research, for the management, integration and analysis of big data of genomic and clinical features, also with the creation of a new language for genomic data management able to express biological queries by means of simple, powerful and orthogonal abstractions. Application to address multiple complex biological questions at the state of the art using publicly available genomic big data.
https://www.bioinformatics.deib.polimi.it/geco/
http://www.bioinformatics.deib.polimi.it/genomic_computing
Funded by: Research Project of National Relevant Interest (PRIN) – Italian Ministry of Education, Universities and Research
Building abstractions, models and protocols for an informatics infrastructure able to support efficiently the organization, search and analysis of a network of genomic data, made available from servers located in the world main biological laboratories
https://gendata.weebly.com/